MSc thesis project proposal
Brain-computer interfaces based on EEG recording of imagined speech
Electroencephalography (EEG) is a noninvasive, low-cost technique widely used to capture the electrical signals generated by neural activity, which can be analyzed to understand both imagined and articulated speech. Classifying imagined speech using EEG is however a challenging task, with classification results close to chance level for systems that are speaker-independent.
To build BCIs that can decode the intended message from neural activity, a thorough understanding of the relationship between neural signals, sounds, articulation, and imagined speech is crucial, and largely lacking.
Recently we recorded a database of EEG recordings from 20 healthy subjects. We collected their EEG during Dutch articulated and imagined speech, recorded in the same trial (see Figure 1), including their speech. We used prompts that consist of five vowels in isolation and as part of 10 consonant-vowel-consonant words, where also the reverse are Dutch words. This allows for the investigation of the neural signatures of vowels in isolation vs. in context, and of consonants in two contexts.
A more thorough check of the database is currently lacking and much needed.
Assignment
In the first part of the thesis project, you will thoroughly analyse the data in order to decipher the neural signatures of (imagined) speech, using well-established signal processing tools. These will include the generation of so-called averaged event-related potentials, event-related synchronization and desynchronization and their comparison across different scenarios both in space and time. Using statistical analysis techniques, you will identify EEG channels and time segments which best distinguish between different vowels and consonants.
Once these distinctive neural signatures are better understood, in the second part of the thesis you will develop a classification pipeline to decode the subject’s brain signals during imagined speech, i.e. to predict consonants, vowels and eventually words that are being imagined at the moment. You will use feature engineering techniques combined with machine learning to develop a pipeline that specifically focuses on the distinctive characteristics of the neural signatures uncovered in the first part.
Requirements
We are looking for students from electrical, biomedical or computer engineering who have experience with signal processing (e.g. statistical signal processing, spectral analysis, spatial filtering) machine learning (e.g. support vector machines, random forest classifiers) and have an interdisciplinary mindset, eager to learn about neuroscience, cognitive science and language representation.
Supervisors: Odette Scharenborg (Multimedia Computing Group), Borbala Hunyadi
Contact
dr. Borbála Hunyadi
Signal Processing Systems Group
Department of Microelectronics
Last modified: 2024-06-28
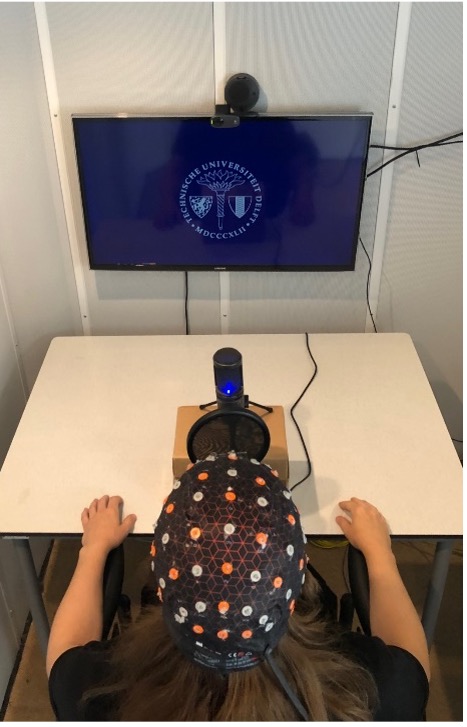